Optimize and Accelerate Machine Learning Inferencing and Training
Speed up machine learning process
Built-in optimizations that deliver up to 17X faster inferencing and up to 1.4X faster training
Plug into your existing technology stack
Support for a variety of frameworks, operating systems and hardware platforms
Build using proven technology
Used in Office 365, Visual Studio and Bing, delivering half Trillion inferences every day
Please help us improve ONNX Runtime by participating in our customer survey.
Get Started Easily
- Optimize Inferencing
- Optimize Training
Platform
Platform list contains six items
Windows
Linux
Mac
Android
iOS
Web Browser (Preview)
API
API list contains eight items
Python
C++
C#
C
Java
JS
Obj-C
WinRT
Architecture
Architecture list contains five items
X64
X86
ARM64
ARM32
IBM Power
Hardware Acceleration
Hardware Acceleration list contains fifteen items
Default CPU
CoreML
CUDA
DirectML
oneDNN
OpenVINO
TensorRT
NNAPI
ACL (Preview)
ArmNN (Preview)
MIGraphX (Preview)
STVM (Preview)
Rockchip NPU (Preview)
Vitis AI (Preview)
Installation Instructions
Please select a combination of resources
Platform
Platform list contains three items
Linux
Windows
Mac
API
API list contains three items
PyTorch 1.8.1
PyTorch 1.9
C++
Architecture
Architecture list contains one item
X64
Hardware Acceleration
Hardware Acceleration list contains four items
Default CPU
CUDA 10.2
CUDA 11.1
ROCm 4.2 (Preview)
ROCm 4.3.1 (Preview)
oneDNN
Installation Instructions
Please select a combination of resources
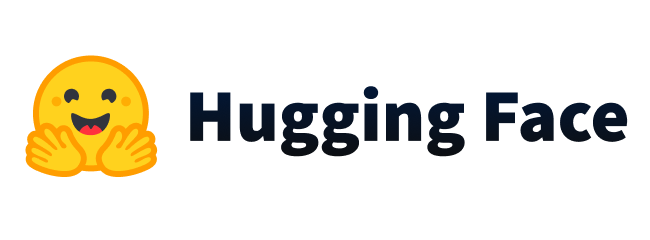
“We use ONNX Runtime to easily deploy thousands of open-source state-of-the-art models in the Hugging Face model hub and accelerate private models for customers of the Accelerated Inference API on CPU and GPU.”
– Morgan Funtowicz, Machine Learning Engineer, Hugging Face
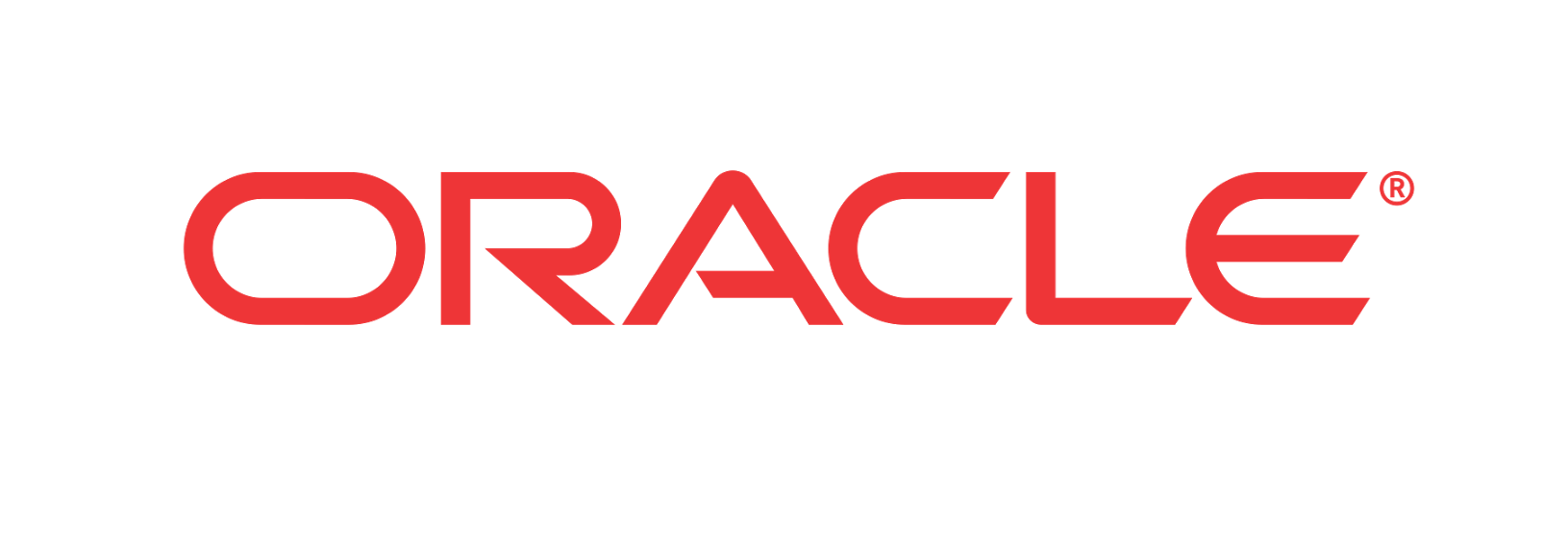
“The ONNX Runtime API for Java enables Java developers and Oracle customers to seamlessly consume and execute ONNX machine-learning models, while taking advantage of the expressive power, high performance, and scalability of Java.”
– Stephen Green, Director of Machine Learning Research Group, Oracle
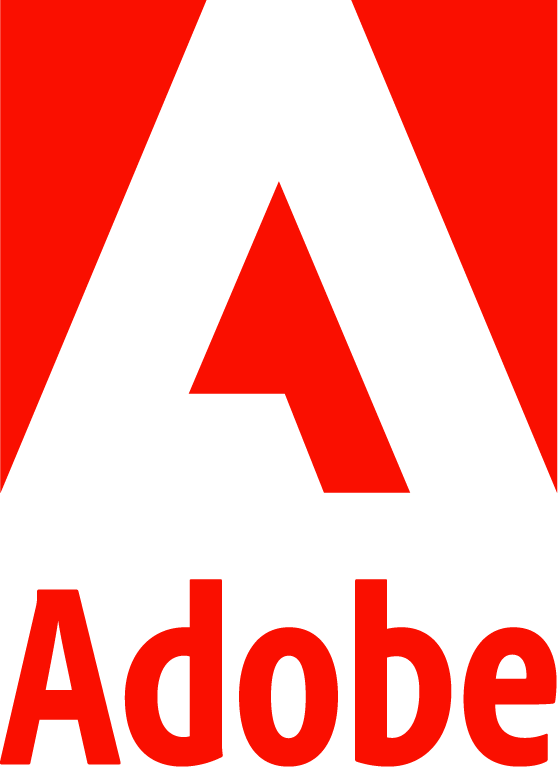
“With ONNX Runtime, Adobe Target got flexibility and standardization in one package: flexibility for our customers to train ML models in the frameworks of their choice, and standardization to robustly deploy those models at scale for fast inference, to deliver true, real-time personalized experiences.”
– Georgiana Copil, Senior Computer Scientist, Adobe
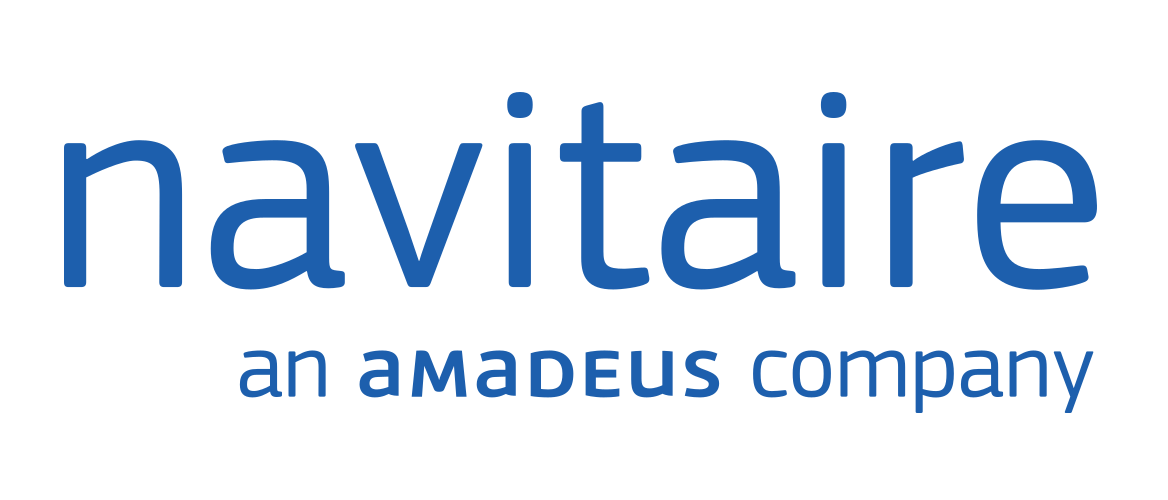
“With customers around the globe, we’re seeing increased interest in deploying more effective models to power pricing solutions via ONNX Runtime. ONNX Runtime’s performance has given us the confidence to use this solution with our customers with more extreme transaction volume requirements.”
– Jason Coverston, Product Director, Navitaire
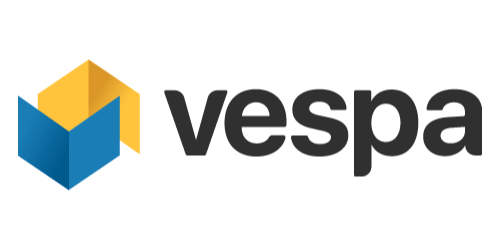
“ONNX Runtime has vastly increased Vespa.ai’s capacity for evaluating large models, both in performance and model types we support.”
– Lester Solbakken, Principal Engineer, Vespa.ai, Verizon Media
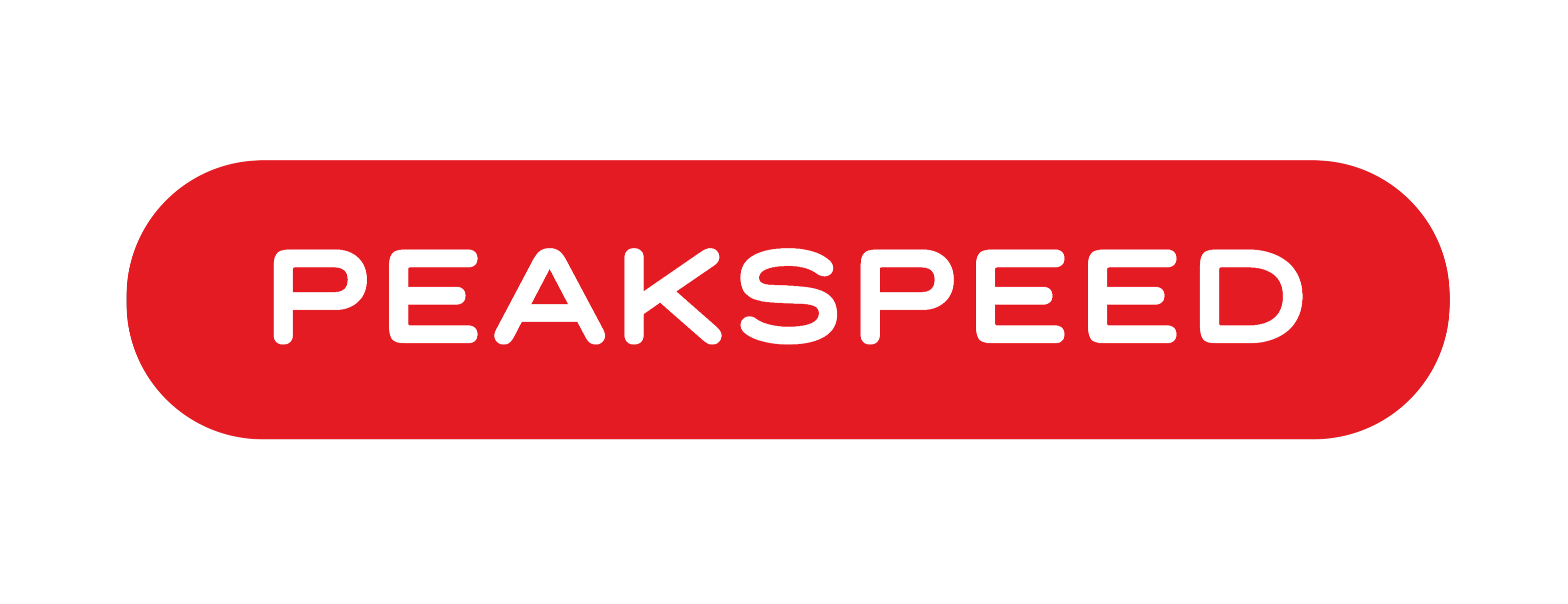
“Using a common model and code base, the ONNX Runtime allows Peakspeed to easily flip between platforms to help our customers choose the most cost-effective solution based on their infrastructure and requirements.”
– Oscar Kramer, Chief Geospatial Scientist, Peakspeed
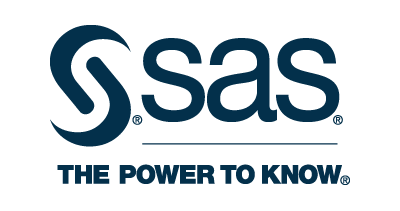
“The unique combination of ONNX Runtime and SAS Event Stream Processing changes the game for developers and systems integrators by supporting flexible pipelines and enabling them to target multiple hardware platforms for the same AI models without bundling and packaging changes. This is crucial considering the additional build and test effort saved on an ongoing basis.”
– Saurabh Mishra, Senior Manager, Product Management, Internet of Things, SAS
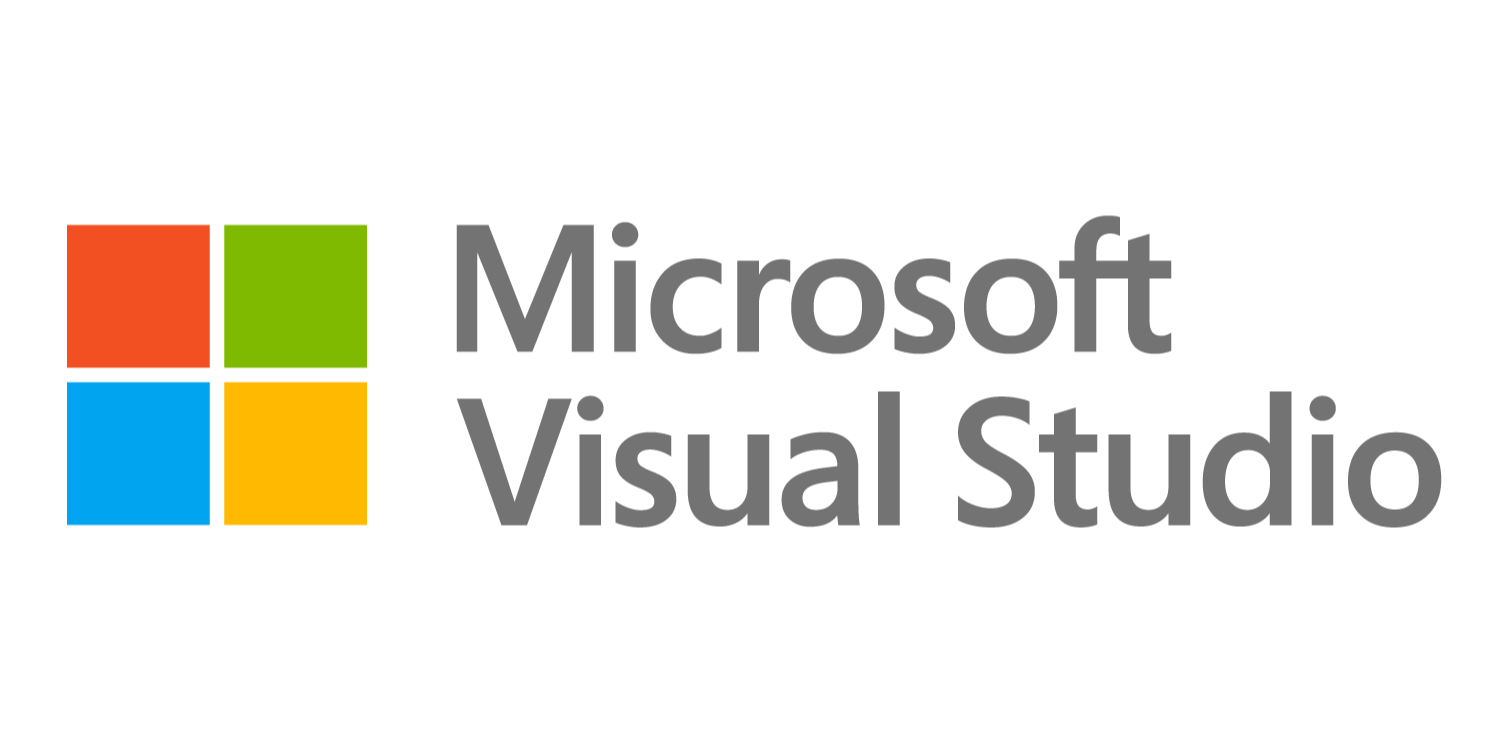
“We use ONNX Runtime to accelerate model training for a 300M+ parameters model that powers code autocompletion in Visual Studio IntelliCode.”
– Neel Sundaresan, Director SW Engineering, Data & AI, Developer Division, Microsoft
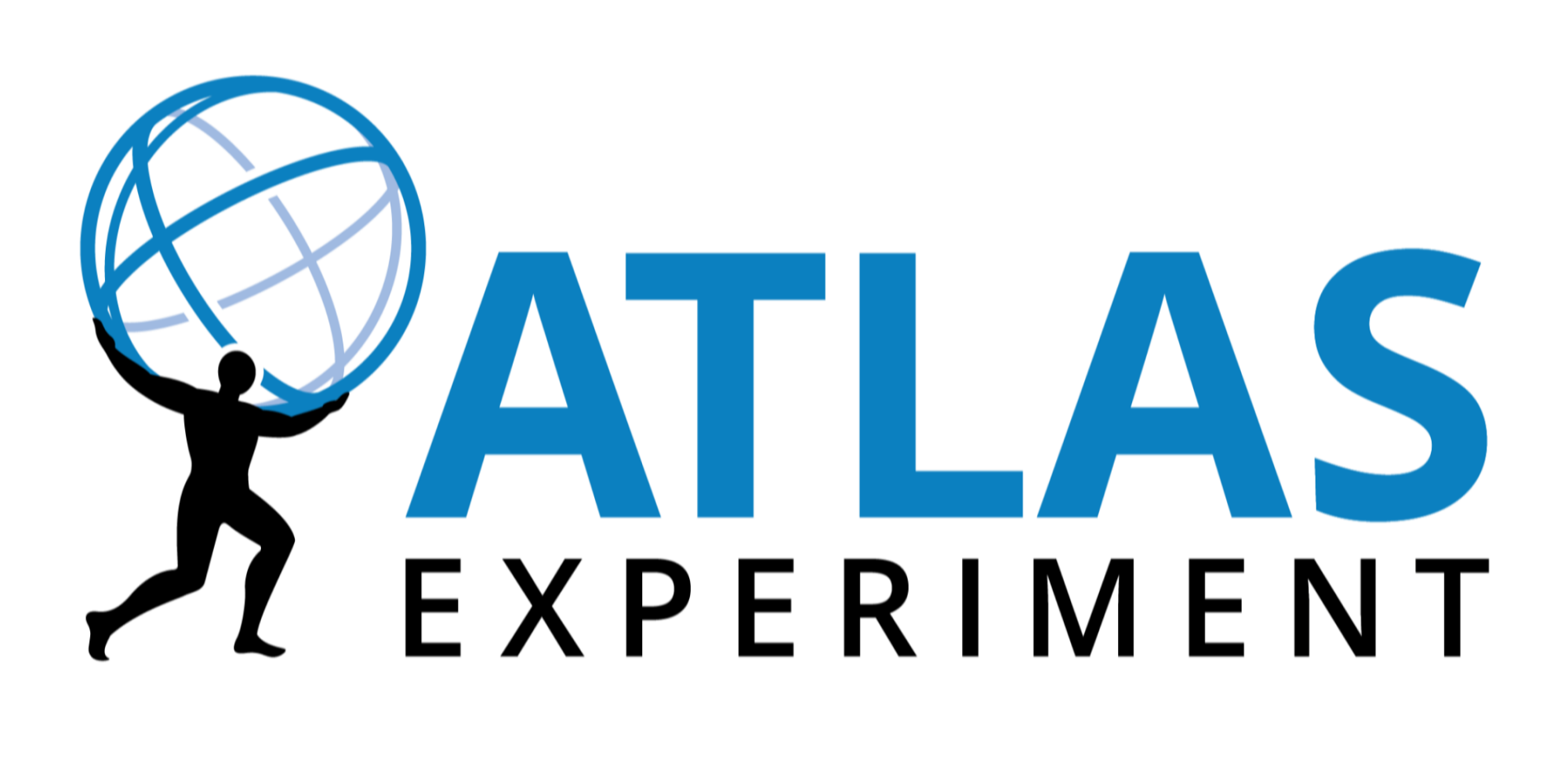
“At CERN in the ATLAS experiment, we have integrated the C++ API of ONNX Runtime into our software framework: Athena. We are currently performing inferences using ONNX models especially in the reconstruction of electrons and muons. We are benefiting from its C++ compatibility, platform*-to-ONNX converters (* Keras, TensorFlow, PyTorch, etc) and its thread safety.”
– ATLAS Experiment team, CERN (European Organization for Nuclear Research)
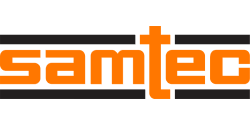
“We needed a runtime engine to handle the transition from data science land to a high-performance production runtime system. ONNX Runtime (ORT) simply ‘just worked’. Having no previous experience with ORT, I was able to easily convert my models, and had prototypes running inference in multiple languages within just a few hours. ORT will be my go-to runtime engine for the foreseeable future.”
– Bill McCrary, Application Architect, Samtec
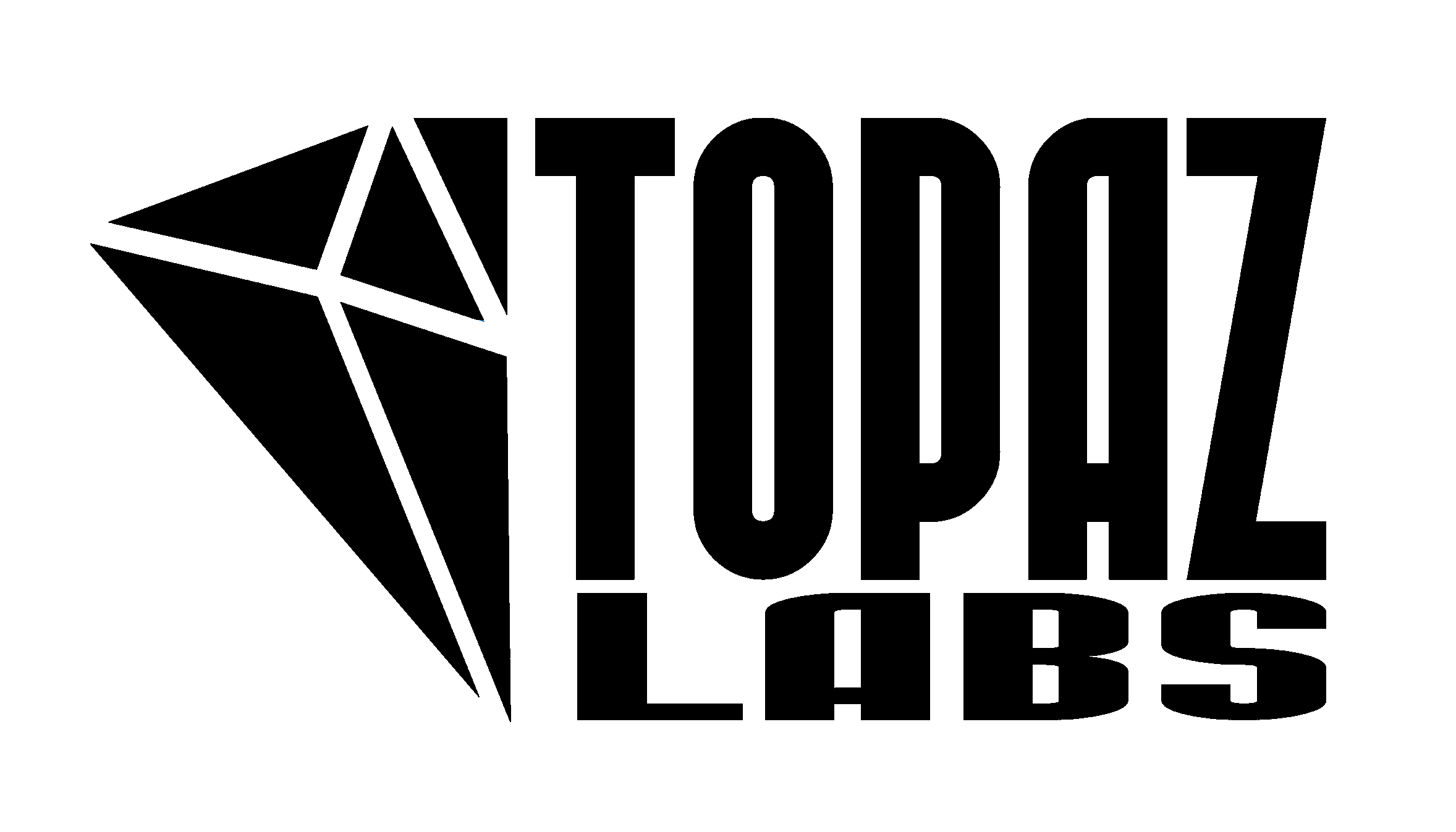
“ONNX Runtime’s simple C API with DirectML provider enabled Topaz Labs to add support for AMD GPUs and NVIDIA Tensor Cores in just a couple of days. Furthermore, our models load many times faster on GPU than any other frameworks. Even our larger models with about 100 million parameters load within seconds.”
– Suraj Raghuraman, Head of AI Engine, Topaz Labs
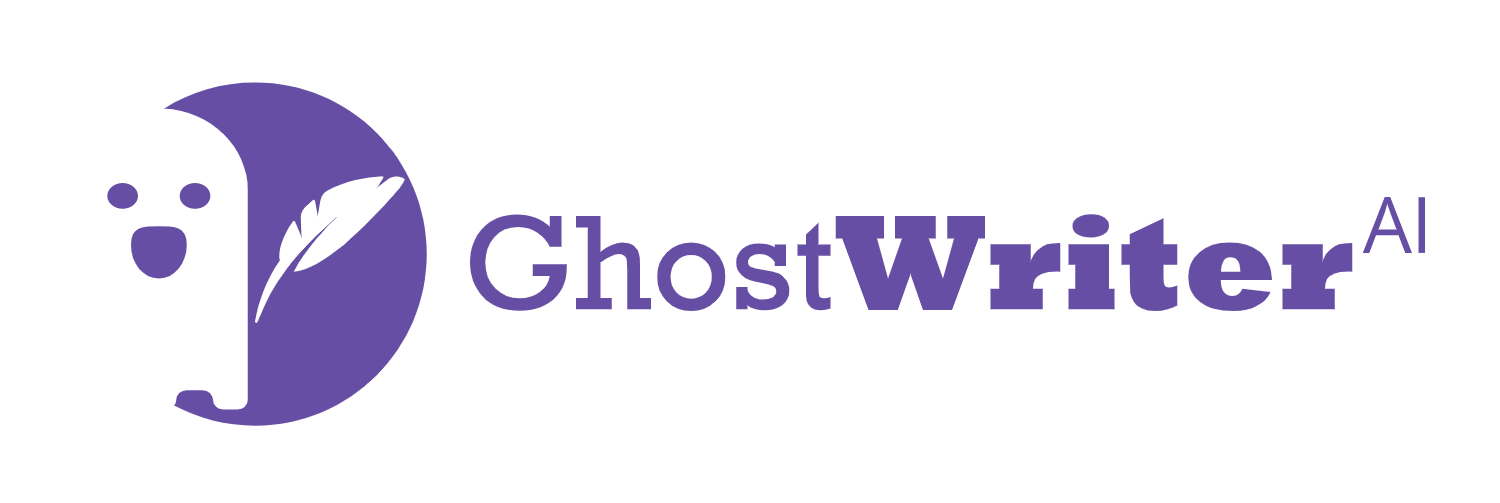
“At GhostWriter.AI, we integrate NLP models in different international markets and regulated industries. Our customers use many technology stacks and frameworks, which change over time. With ONNX Runtime, we can provide maximum performance combined with the total flexibility of making inferences using the technology our customers prefer, from Python to C#, deploying where they choose, from cloud to embedded systems.”
– Mauro Bennici, CTO, Ghostwriter.AI
News & Announcements
Accelerate PyTorch transformer model training with ONNX Runtime – a deep dive
ONNX Runtime (ORT) for PyTorch accelerates training large scale models across multiple GPUs with up to 37% increase in training throughput over PyTorch and up to 86% speed up when combined with DeepSpeed...Read more
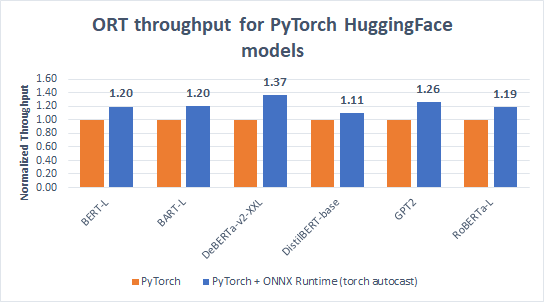
Accelerate PyTorch training with torch-ort
With a simple change to your PyTorch training script, you can now speed up training large language models with torch_ort.ORTModule, running on the target hardware of your choice...Read more
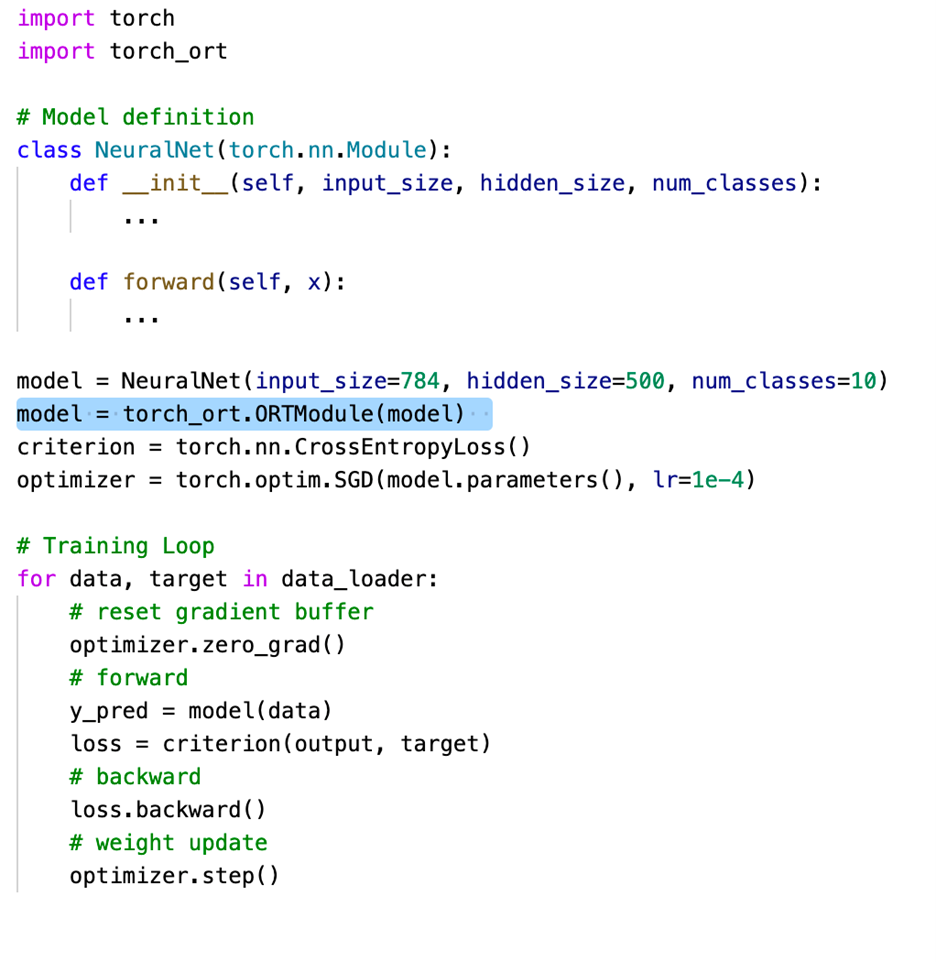
Journey to optimize large scale transformer model inference with ONNX Runtime
Large-scale transformer models, such as GPT-2 and GPT-3, are among the most useful self-supervised transformer language models for natural language processing tasks such as language translation, question answering, passage summarization, text generation, and so on...Read more
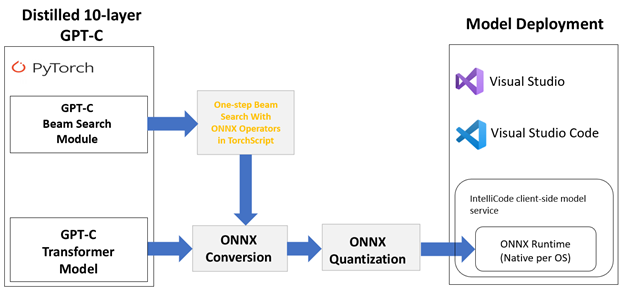
ONNX Runtime release 1.8.1 previews support for accelerated training on AMD GPUs with the AMD ROCm™ Open Software Platform
ONNX Runtime is an open-source project that is designed to accelerate machine learning across a wide range of frameworks, operating systems, and hardware platforms. Today, we are excited to announce a preview version of ONNX Runtime in release 1.8.1 featuring support for AMD Instinct™ GPUs facilitated by the AMD ROCm™ open software platform...Read more

SAS and Microsoft collaborate to democratize the use of Deep Learning Models
Artificial Intelligence (AI) developers enjoy the flexibility of choosing a model training framework of their choice. This includes both open-source frameworks as well as vendor-specific ones. While this is great for innovation, it does introduce the challenge of operationalization across different hardware platforms...Read more
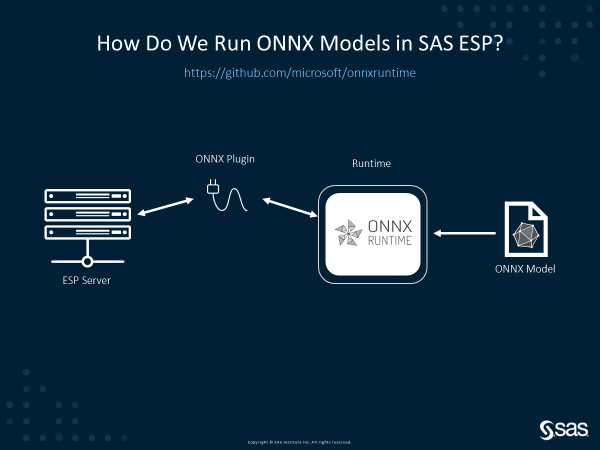
Optimizing BERT model for Intel CPU Cores using ONNX runtime default execution provider
The performance improvements provided by ONNX Runtime powered by Intel® Deep Learning Boost: Vector Neural Network Instructions (Intel® DL Boost: VNNI) greatly improves performance of machine learning model execution for developers...Read more
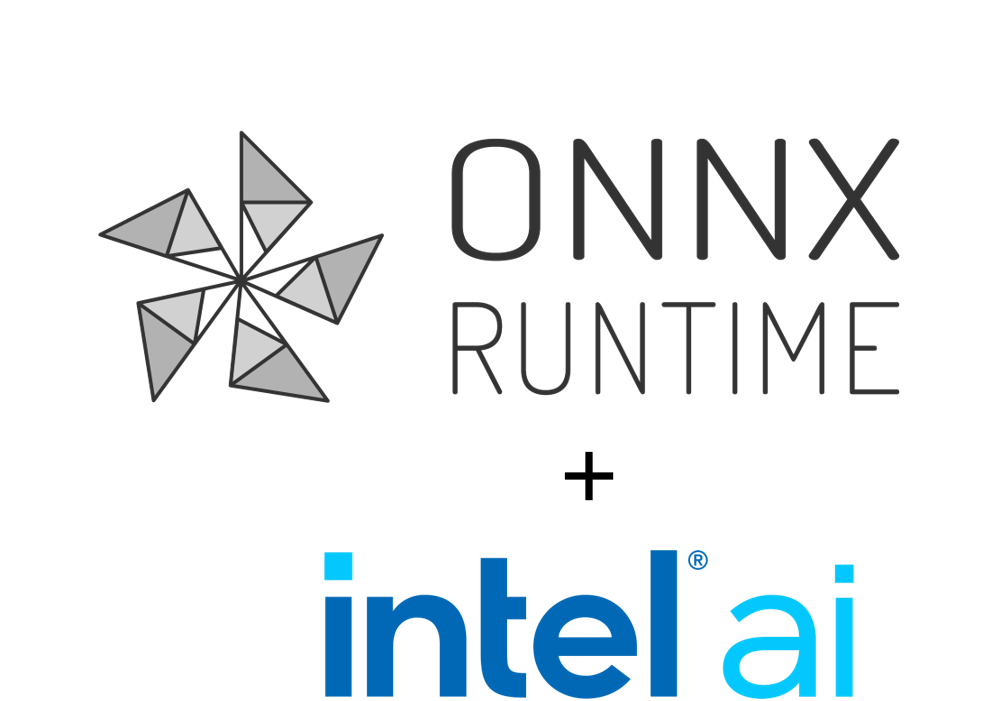
Resources
Hardware Ecosystem
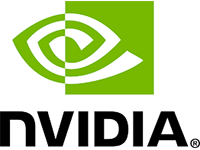
“ONNX Runtime enables our customers to easily apply NVIDIA TensorRT’s powerful optimizations to machine learning models, irrespective of the training framework, and deploy across NVIDIA GPUs and edge devices.”
– Kari Ann Briski, Sr. Director, Accelerated Computing Software and AI Product, NVIDIA
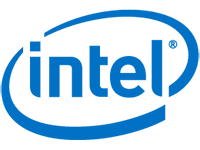
“We are excited to support ONNX Runtime on the Intel® Distribution of OpenVINO™. This accelerates machine learning inference across Intel hardware and gives developers the flexibility to choose the combination of Intel hardware that best meets their needs from CPU to VPU or FPGA.”
– Jonathan Ballon, Vice President and General Manager, Intel Internet of Things Group
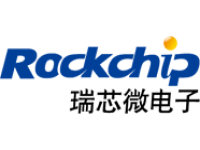
“With support for ONNX Runtime, our customers and developers can cross the boundaries of the model training framework, easily deploy ML models in Rockchip NPU powered devices.”
– Feng Chen, Senior Vice President, Rockchip
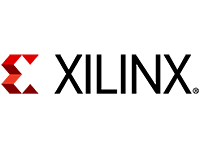